Balancing Innovation and Practicality in AI Projects: A Guide for IT Leaders
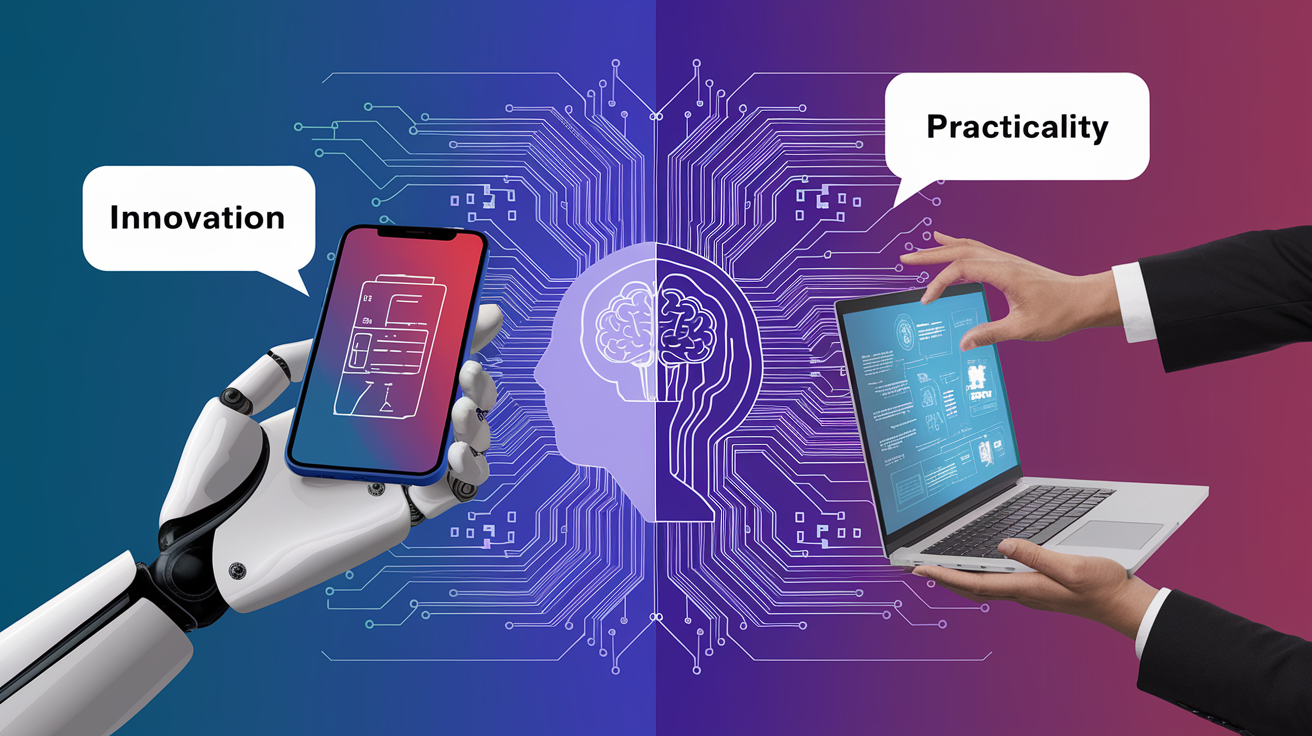
As artificial intelligence (AI) continues to evolve at a rapid pace, IT leaders face the challenge of harnessing its innovative potential while ensuring practical, value-driven implementations. Striking the right balance between cutting-edge AI capabilities and practical business solutions is crucial for successful AI adoption. This article provides guidance for IT leaders on how to navigate this balance, ensuring AI projects deliver both innovation and tangible business value.
The Innovation-Practicality Dilemma
The field of AI is characterized by constant innovation, with new models, techniques, and applications emerging regularly. However, businesses need solutions that are:
- Reliable and stable
- Cost-effective
- Aligned with existing processes and systems
- Compliant with regulations
- Accessible to end-users
Balancing these practical concerns with the desire to leverage the latest AI innovations can be challenging.
Key Considerations for Balancing Innovation and Practicality
1. Align AI Initiatives with Business Objectives
- Start with clear business goals rather than specific AI technologies
- Evaluate how AI can address existing pain points or create new opportunities
- Ensure AI projects have a clear path to ROI
2. Assess Organizational Readiness
- Evaluate existing infrastructure, data quality, and team capabilities
- Consider the cultural readiness for AI adoption
- Identify any regulatory or compliance constraints
3. Prioritize Use Cases
- Focus on high-impact, feasible projects first
- Consider both short-term wins and long-term transformational projects
- Balance risk and reward in your AI project portfolio
4. Choose the Right Level of AI Sophistication
- Not every problem requires the most advanced AI solution
- Consider simpler, rules-based automation for straightforward tasks
- Reserve cutting-edge AI for complex problems where it can provide significant value
5. Build with Scalability in Mind
- Design AI solutions that can grow with your organization
- Consider how today's projects can lay the groundwork for future innovations
- Implement modular architectures that allow for easy updates and expansions
6. Emphasize Data Quality and Governance
- Prioritize data cleaning, integration, and governance
- Implement robust data pipelines to ensure consistent, high-quality inputs for AI systems
- Consider privacy, security, and ethical implications of data usage
7. Foster Cross-functional Collaboration
- Involve both technical teams and business stakeholders in AI projects
- Encourage knowledge sharing between data scientists, domain experts, and end-users
- Create multidisciplinary teams to ensure well-rounded solution design
8. Implement Agile and Iterative Approaches
- Start with minimum viable products (MVPs) and iterate based on feedback
- Use agile methodologies to adapt quickly to changing requirements or new innovations
- Continuously evaluate and refine AI models based on real-world performance
9. Invest in Change Management and Training
- Prepare your workforce for AI adoption through comprehensive training programs
- Communicate the benefits and limitations of AI systems clearly
- Address concerns and resistance proactively
10. Stay Informed About AI Advancements
- Keep abreast of the latest AI research and industry trends
- Attend conferences, webinars, and workshops to stay updated
- Consider partnerships with academic institutions or AI research organizations
Case Study: Balancing Innovation and Practicality in Customer Service AI
Let's consider a hypothetical case study to illustrate these principles in action:
Company: A mid-sized e-commerce retailer Objective: Improve customer service efficiency and satisfaction
Approach:
- Assessing Needs: The company identified long response times and inconsistent service quality as key pain points.
- Choosing Technology: Rather than immediately opting for the most advanced conversational AI, they decided on a phased approach:
- Phase 1: Implement a rule-based chatbot for handling common queries
- Phase 2: Introduce an AI-powered sentiment analysis tool for prioritizing critical customer issues
- Phase 3: Develop a more sophisticated AI chatbot using natural language processing
- Implementation:
- Started with a simple chatbot that could handle 50% of common customer queries
- Integrated the chatbot with existing customer service systems
- Trained customer service representatives to work alongside the AI system
- Iteration and Scaling:
- Gathered user feedback and chatbot interaction data
- Gradually expanded the chatbot's capabilities based on real-world performance
- Introduced sentiment analysis to help human agents prioritize urgent cases
- Results:
- 30% reduction in average response time
- 25% increase in customer satisfaction scores
- Successful adoption by customer service team, with AI handling 70% of initial customer contacts
This phased approach allowed the company to balance innovation with practicality, delivering immediate benefits while laying the groundwork for more advanced AI implementations in the future.
Overcoming Common Challenges
- Overhyped Expectations: Be realistic about what AI can achieve in the short term. Educate stakeholders about both the potential and limitations of AI.
- Resistance to Change: Involve end-users in the design process and clearly communicate how AI will support (not replace) their work.
- Data Silos and Quality Issues: Invest in data integration and cleaning efforts early in the project. Implement robust data governance practices.
- Lack of Skilled Personnel: Consider partnering with AI consultants or service providers. Invest in training programs to upskill existing staff.
- Ethical and Bias Concerns: Implement ethics guidelines for AI development and usage. Regularly audit AI systems for bias and unintended consequences.
The Road Ahead: Embracing Continuous Innovation
As AI continues to evolve, maintaining the balance between innovation and practicality will be an ongoing process. IT leaders should:
- Establish an AI Center of Excellence to centralize knowledge and best practices
- Create a culture of experimentation and learning from failures
- Develop partnerships with AI vendors, researchers, and academic institutions
- Implement robust governance frameworks to ensure responsible AI adoption
- Regularly reassess and adjust AI strategies to align with evolving business needs and technological capabilities
Conclusion
Balancing innovation and practicality in AI projects is not about choosing one over the other, but rather finding the sweet spot where cutting-edge technology meets real-world business needs. By taking a thoughtful, strategic approach to AI adoption, IT leaders can drive meaningful innovation while ensuring their projects deliver tangible value.
The key lies in starting with clear business objectives, choosing the right level of AI sophistication for each use case, and implementing solutions in an iterative, scalable manner. By fostering a culture of continuous learning and adaptation, organizations can stay at the forefront of AI innovation while maintaining a strong focus on practical, value-driven implementations.
For IT leaders navigating the complex landscape of AI, the goal should be to create a portfolio of AI initiatives that balance quick wins with transformational projects, always keeping an eye on both the technological horizon and the immediate needs of the business. With this balanced approach, organizations can harness the full potential of AI to drive growth, efficiency, and competitive advantage in the digital age.